Data Preprocessing: An Essential Step to Knowledge Discovery
- Rosmina Joy Cabauatan
- Mar 24, 2021
- 4 min read
Updated: Apr 2, 2021
We are living in an age where we are deluged by data. The irrepressible growth of these data naturally evolved due to the utilization of information technology and the digital transformation of society. The demand for more advanced systems with increased storage capacities and processing power has contributed to the growth and availability of data. These developments have influenced more effective mechanisms for data storage, retrieval, and data processing, which resulted in the generation of voluminous data. These data eventually became a valuable resource that aids decision-making. As an economic asset, it helps institutions improve operations, increase revenue, improve the quality of products and services, and remain competitive. Undeniably, data is an asset that can leverage digital presence.
Data can be collated from different sources and their natural forms challenge the data analysis. It is at this premise, that data cleaning becomes an essential step of discovering knowledge from data. Some of the challenges are commonly referred to as the 4Vs of data. The following figure shows these challenges.
4Vs of Data
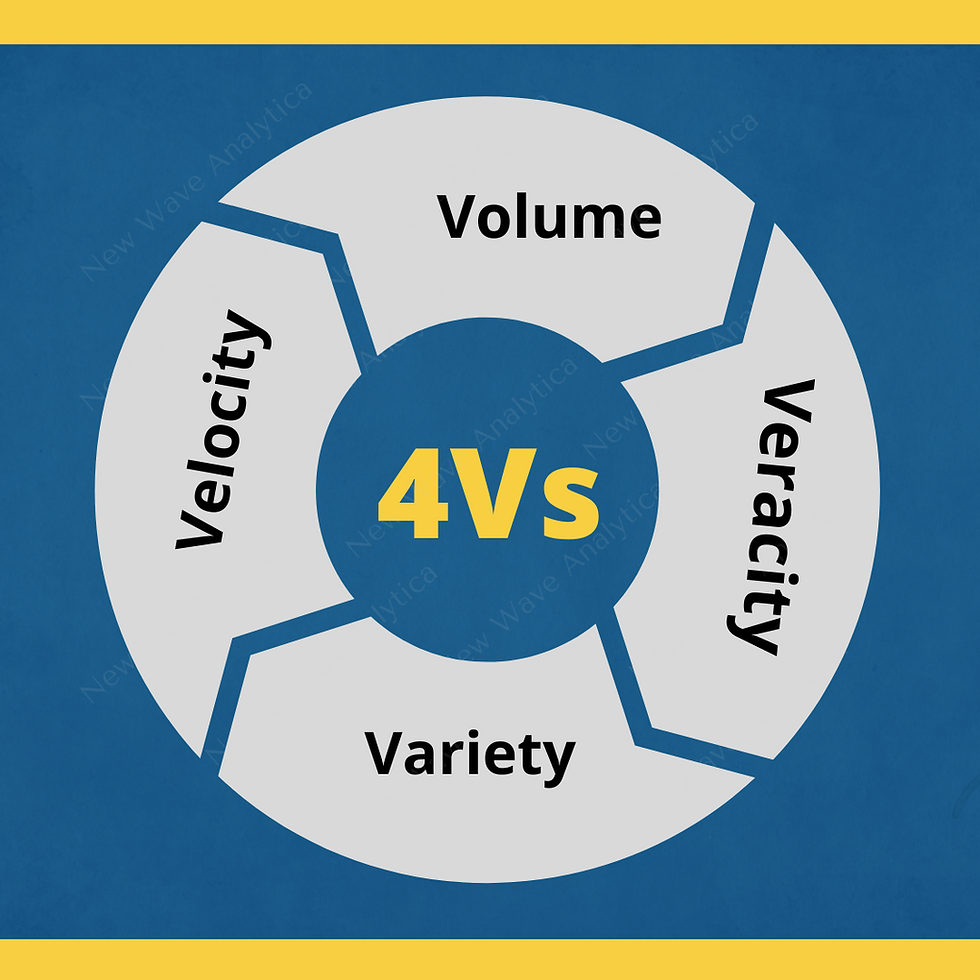
Volume describes the challenge of storing and processing the enormous quantity of collected data. As data collection increases, it is more difficult to find the correct data and slows down the processing speed of data analysis tools. It is expected that data be increased 400 times from 2018 or roughly 50 zettabytes in the next 2 years.
Variety encompasses the many different types of data that are collected and stored. Data analysis tools must be equipped to simultaneously process a wide array of data formats and structures.
Velocity details the increasing speed at which new data is created, collected, and stored. Because of data velocity, data analysis is challenged in associating the rapidly increasing rate of data generation.
Veracity acknowledges that not all data is equally accurate. Data can be messy, incomplete, improperly collected, and even biased. The quicker the data is collected, the more errors will manifest within the data. The challenge of veracity is to balance the quantity of data with its quality.
Data Preprocessing
Real-world data are susceptible to noise and inconsistencies, incomplete, and have many missing components. So how can data be preprocessed to help improve the quality of data analysis results? How can the data be preprocessed to enhance the efficiency and ease of data analysis?
Several techniques can be applied to perform data preprocessing. Some of these techniques are as follows:
Data cleaning can be applied to remove noise and correct inconsistencies in data.
Data integration merges data from multiple sources into coherent data storage such as data warehouses.
Data reduction can reduce the size by, for instance, aggregating, eliminating redundant features, or scaled to fall within a smaller range.
These techniques can improve the accuracy and efficiency of algorithms used for data analysis. The methods are not mutually exclusive; they can work together.
Why Preprocess Data?
Data has quality if it satisfies the requirements of the intended use. The following are the factors comprising data quality.
accuracy
completeness
consistency
timeliness
believability
interpretability
Among these factors, the most common that can compromise data quality in large data sets are accuracy, completeness, and consistency.
There are many possible reasons for inaccurate data. Some of these are incorrect attribute values, faulty data collection tools, human or computer errors at data entry, users may purposely submit incorrect personal information, and technology limitations. These reasons result in data inconsistencies. Incomplete data can occur for several reasons such as unavailability of data attributes of interest, malfunctioning equipment, some data may not be recorded because they are not considered necessary at the time of entry. Data that were inconsistent with other recorded data may have been deleted. Recoding of the data history or modifications may have been overlooked.
Major Tasks of Data Preprocessing

Data Cleaning
Real-world data tend to be dirty. Data preprocessing is an essential step in the knowledge discovery process because quality decisions must be based on quality data. Detecting and rectifying them early, and reducing the data to be analyzed can lead to enormous payoffs for decision making.
Data Integration
Data integration is the merging of data from multiple data storage. Careful integration can help avoid redundancies and inconsistencies in the resulting data set. This can help improve the accuracy and speed of the subsequent data mining process.
Data Reduction
Data reduction is applied to data to obtain a reduced representation of the data set that is much smaller in volume, yet closely maintains the integrity of the original data. That is, mining on the reduced data set should be more efficient yet produce the same or almost the same results.
Data Transformation
Data transformation is a function that maps the entire set of values of a given attribute to a new set of replacement values such that. each old value can be identified with one of the new values.
After knowing the value of data and the significance of data processing, are you now ready to discover knowledge from data?
Leverage your digital presence,
New Wave Analytica provides data analytics services.

Contact Us
Quezon City, Philippines Tel. Nos. (+632) 9369576958, +639619811097 E-mail: joyc.itfreelancejobs@gmail.com
New Wave Analytica is also affiliated to Analytica Learning Academy, an institution that is offering data analytics and IT courses.
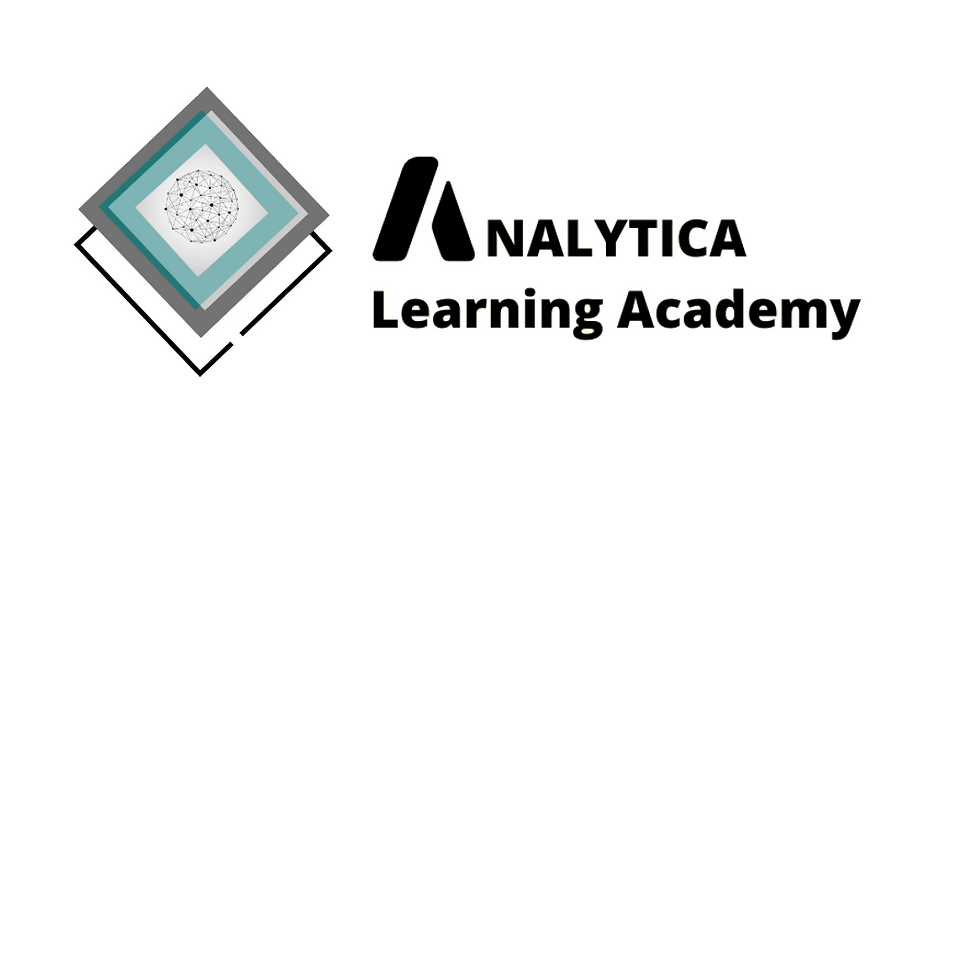
Comments